How do AI death calculators work? Artificial intelligence (AI) powered death calculators have become increasingly popular in recent years. These online tools use machine learning algorithms to estimate a person’s remaining life expectancy based on various factors like age, lifestyle, family history, etc. In this comprehensive guide, we will explore how these futuristic AI-driven mortality predictors work and look under the hood at the technology powering them.
What are AI death calculators?
AI death calculators are automated online tools that leverage large datasets and machine learning techniques to predict an individual’s risk of dying within a given timeframe, such as the next 5 or 10 years. Some of the popular AI death calculators available today include:
- Lifespan Calculator by Lifespan.io
- Death Clock by Death Clock
- How Long Will I Live? by LivingTo100
- Death Date Calculator by Death Date
These use self-reported information like age, smoking habits, medical history, family health, etc. to generate personalized mortality estimates. The risk evaluation methodology used by each calculator varies but AI and neural networks play an important role in crunching the data to output survival probabilities.
Why estimate mortality risk?
Predicting life expectancy and risk of death serves many purposes:
- It helps people assess lifestyle risks and make appropriate changes if required. Knowing one’s risk of dying from preventable causes can motivate positive behavior modification.
- Doctors use risk calculators to screen patients, customize treatment plans, and recommend preventative healthcare strategies. Predictive analytics improves clinical decision making.
- For insurance companies, accurate mortality estimates help price policies and manage profitability. AI is transforming the way life insurance is underwritten.
- Public health agencies rely on death risk analytics to study population health trends and craft targeted interventions. It supports evidence-based policymaking.
- Researchers use survival probability models to investigate factors affecting longevity and causes of premature death. It provides insights to extend lifespan.
By forecasting end-of-life dates, death calculators promote risk awareness and longevity. But their predictions are only as good as the algorithms behind them.
Data sources used for analysis
The mortality risk assessments generated by AI death calculators are powered by machine learning algorithms. The most critical ingredient that determines their accuracy is the training data. Here are the key data sources leveraged:
Population databases
Anonymous population data about age, gender, ethnicity, education, income, marital status, etc. provide the demographic foundation. The US Census and survey data from government agencies like CDC and NCHS are commonly used. These capture population-level traits affecting average life expectancy.
Medical studies
Clinical research data linking risk factors like smoking, obesity, inactivity, hypertension to mortality outcomes are used. Long term public health studies like Framingham Heart Study that have tracked health cohorts for decades contribute crucial data.
Insurance records
Anonymous databases containing insurance purchase history, family medical records, prescription drug information, and hospitalization data provide further individuating signals. This real-world data improves risk models.
User inputs
Personal data like age, gender, family history, lifestyle that users provide while using the calculator adds customization. Information given voluntarily fine tunes the prediction to the individual.
High-quality datasets gathered across large diverse populations over long time periods allow creating sophisticated AI models that generate reliable mortality risk estimates.
How AI algorithms calculate death risk
Here are the key techniques used by AI death calculators to process data and estimate survival probabilities:
Lifetable analysis
This estimates population-level mortality rates from census and demographic data. The death rate for each age group in the population is calculated to create a mortality curve. This establishes an average baseline.
Cox proportional hazards
This statistical method identifies risk factors from clinical data and calculates the hazard ratio for each i.e. how much it raises/reduces mortality. Powerful in analyzing time-to-death data from cohort studies.
Random survival forests
An ensemble machine learning technique that builds multiple decision trees to model survival data. Each tree uses a random subset of features to make survival estimates. This overcomes overfitting.
Neural networks
Deep learning algorithms discern complex nonlinear patterns between input health parameters and survival outcomes. Neural nets find interactions between variables missed by traditional techniques.
Natural language processing
NLP techniques analyze text data from health questionnaires and electronic health records to extract risk signals. Unstructured medical data contains insights that improve predictions.
The outputs of the above techniques are blended using statistical meta-learning algorithms to generate individual risk forecasts. The AI models are recalibrated as new data comes in. By combining computer science and medical science, they keep improving their accuracy.
Challenges in estimating death risk
Despite significant progress, AI-powered death prediction still faces some challenges:
- Human lifetimes are highly variable and lifespan correlates are still being studied. Cause-effect models of mortality are limited.
- Quality longitudinal health data across diverse populations is lacking. Training data deficiencies create bias.
- Medical science evolves continuously. Risk models need constant updating to factor in new health knowledge.
- Not all risk variables can be known. Individuals may have undisclosed predispositions or habits affecting health.
- Humans can deliberately change behavior and override risk expectations. Free will makes risk estimation inherently fuzzy.
- Explaining AI model decisions for transparency and accountability remains difficult.
- Predicting remaining life expectancy versus estimating exact death dates are fundamentally different problems. Most calculators do the former.
To summarize, death calculators rely on big data and AI to assess mortality risk. But human lifespan has many uncontrollable complexities that limit fate prediction. The algorithms simplify reality and cannot foresee all factors. Hence users should see risk estimates as informed approximations rather than incontrovertible prophesies.
Use case examples
Here are some examples demonstrating the utility of AI-powered death risk estimators:
- A 45-year obese smoker sees a 60% risk of dying in the next 10 years. This prompts joining a gym, quitting smoking, and scheduling medical tests. Early intervention improves prognosis.
- A primary care doctor uses an AI tool that highlights a 52-year patient’s risk of cardiovascular death is in the danger zone due to hypertension. The doctor adjusts medications and monitors heart health.
- A life insurance company uses a deep learning model which combines demographics, biometric data, and consumer purchase history to customize premiums based on mortality risk scores.
- Public health bodies draw insights from AI to segment populations, identify high-risk subgroups, and drive targeted health campaigns e.g. promoting cancer screening in certain geographic areas.
- Genetic analysis reveals a mutation increasing Alzheimer’s risk in a 40-year person’s family. An AI tool estimates onset age as 73 years. The person enrolls in a clinical trial for an experimental drug.
The applications clearly demonstrate how AI mortality estimates enable data-driven decisions across industries, communities, and individuals – ultimately benefiting human health and longevity.
Conclusion
In this digital era, AI is transforming simplicity predicting. Death calculators combine big data, medical science and machine learning to generate personalized mortality risk estimates. These interactive tools help people evaluate end-of-life risks and take appropriate actions. However, their predictions have limitations due to the complexity of human longevity.
Looking ahead, with larger datasets, better modelling techniques and advances in medical science, the accuracy of AI death risk estimators will continue improving. But human lifespan has an inherent unpredictability that technology cannot fully eliminate. At best, AI calculators can provide reasonable risk guidance by approximating the incredibly complex dynamics affecting length of life.
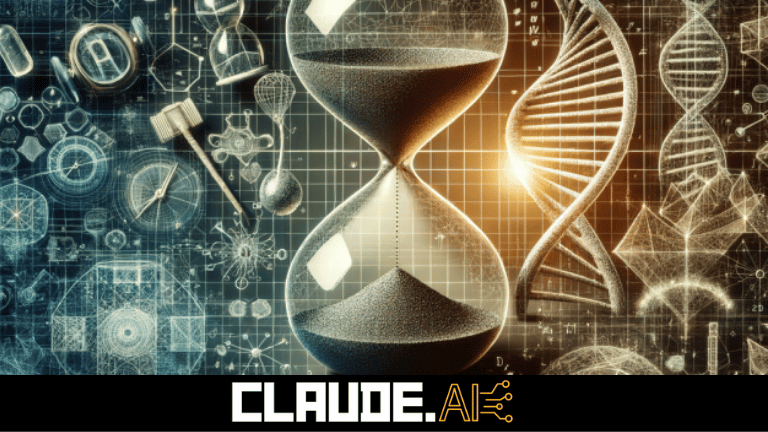