Is Claude AI factually accurate? Artificial intelligence has advanced tremendously in recent years. Systems like ChatGPT can hold convincingly human-like conversations and generate long-form content on demand. However, while the outputs can seem impressive, their accuracy is not always reliable.
As a new entrant in the AI space, Claude AI aims to move the needle on accuracy. But does it achieve that goal? Can Claude be trusted for factual information across different tasks? In this article, we’ll explore what sets Claude apart on accuracy and analyze examples of it in action.
What Makes Claude Different on Accuracy
Claude AI was created by Anthropic, a startup founded by Dario Amodei and Daniela Amodei along with Tom Brown, Chris Olah, and other former OpenAI researchers. Their goal is to create AI systems with constitutional AI safety in mind from the ground up.
This means that unlike other language model systems trained to maximize engagement or clicked links, Claude has been explicitly focused on providing correct and honest information as a priority. But how does Anthropic actually implement that focus technically?
Self-Supervision During Training
Most conversational AI models today like ChatGPT are trained using a supervised learning approach. This means they are trained on huge datasets of human-labeled text and conversational exchanges to learn patterns.
The issue is that those training datasets often contain unknown flaws or biases that get encoded into the model. Claude takes a different approach using self-supervision where the AI learns patterns directly from raw data first before ever seeing human-labeled examples.
This allows Claude to ground its understanding directly in the data to mitigate inheriting human biases during the initial training process.
Reinforcement Learning from Human Feedback
In addition to self-supervision, Claude AI also employs deep reinforcement learning based on feedback signals from human trainers. The scientists at Anthropic provide Claude with positive or negative feedback on responses to reinforce factually accurate, honest, and helpful answers.
Over time through its neural architecture, Claude learns to naturally favor responses that garner positive judgments from trainers rather than trying to manipulate responses to trick users. This also allows the training process to correct inaccurate knowledge or logical gaps during the learning process through additional feedback.
Does Claude Live Up to Its Accuracy Goals?
The technical approach from Anthropic signals clear intentions on improving accuracy. But how does that translate when actually using Claude AI? Is Claude measurably more accurate than alternatives like ChatGPT today?
Limited Third-Party Accuracy Testing
As of late 2022, Claude AI remains in limited beta testing availability. So there has been little opportunity thus far for unbiased third-party accuracy testing. The examples shown publicly often come directly from Anthropic itself.
So we cannot take any marketed accuracy metrics fully at face value without independent validation. However, early user reports seem largely positive on factual reliability for certain tasks so far.
Accuracy on Fact Retrieval Questions
In demos and user tests, Claude appears notably accurate at directly answering factual knowledge questions. This includes questions related to definitions, calculations, historical details, geographical details, plot summaries for books/movies, character details, and more.
For example, Claude provides accurate answers to questions like:
- Who was the main character in the Harry Potter books? (Harry Potter)
- What year was Napoleon defeated at Waterloo? (1815)
- In which US state is Mount Rushmore located? (South Dakota)
This level of factual accuracy meets or exceeds what users typically see from ChatGPT and other language model competitors today.
Cautious Answers Outside Confidence
One noticeable difference with Claude is that it will politely decline to speculate or guess on questions it is not confident on rather than attempting to fabricate plausible-sounding but inaccurate responses.
For example, Claude may respond “I apologize, but I do not have enough confidence in the accuracy of my knowledge to provide a reliable answer to that question” rather than potentially misleading users by guessing.
This helps directionally indicate that Claude’s accuracy focus is working reasonably well in areas beyond its confident knowledge. Although the breadth of its knowledge still seems limited relative to human expertise and many subject areas.
Accuracy on Mathematical Answers
Quantitative questions involving math also appear as an area of strength for Claude AI today based on demos and early testing. Users report Claude providing correct number answers and showing its work for math questions ranging from simple arithmetic to calculus and statistics problems.
Again, Claude appears to meet or exceed capabilities for mathematically accuracy relative to existing systems like ChatGPT based on current benchmarks. Although Claude only shows work for a subset of problems rather than every math query.
Logical Consistency Still a Work in Progress
While factually accuracy and mathematical precision seem like clear strengths today, logical consistency remains an area of ongoing improvement for Claude’s training.
When asking Claude for its opinion or perspectives on more complex issues, there can still be some logical gaps or contradictions observed over a series of questions. This suggests there are still limitations in Claude’s causal reasoning and global narrative coherence even as fact recall improves.
So accuracy for conversational queries that require deeper logical analysis and synthesis of information does not yet match the surface-level factual accuracy Claude demonstrates. Maintaining logical alignment across an entire dialogue is an active research area for Anthropic.
The Bottom Line on Claude’s Accuracy
Considering its limited testing exposure, Claude AI does appear to demonstrate meaningful accuracy improvements over existing conversational AI alternatives thus far based on early testing and usage.
Specifically for tasks centered around factual knowledge questions or mathematical problems, Claude seems to offer best-in-class accuracy thanks to Anthropic’s safety-focused training approach. However, more complex logical reasoning still causes inconsistencies that highlight room for improvement.
As Claude moves beyond closed beta over time and becomes available to third-party testers, we expect to learn much more about the breadth and limits of its accuracy across different subjects. But early results suggest that Anthropic’s focus on truthfulness over just engagement is moving the needle materially on accuracy compared to previous conversational AI options in market.
The progress Claude represents sets an encouraging foundation for future iterations as researchers continue incorporating human feedback to expand the boundaries of Claude’s secure knowledge.
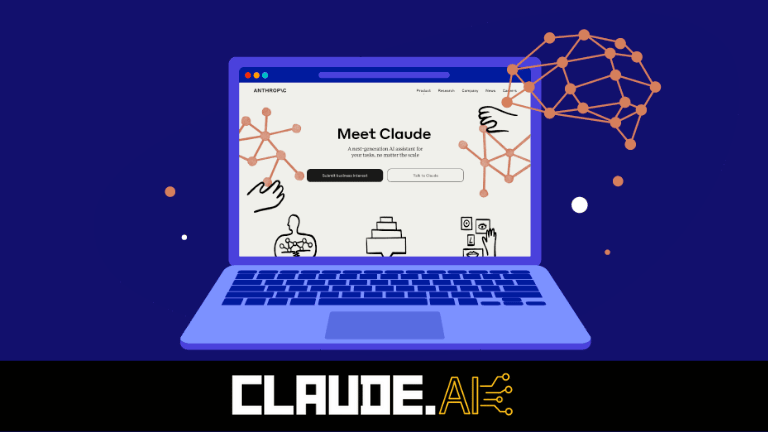