How Does GPT-4’s Development Differ from Claude 2.1? OpenAI’s GPT-4 and Anthropic’s Claude 2.1 are two of the most advanced conversational AI systems developed to date. They represent different yet equally promising paths towards advanced artificial general intelligence (AGI).
While they share some technological similarities in their use of transformer-based neural networks, their underlying architectures, training methodologies, and intended use cases are quite different. Understanding how GPT-4 and Claude 2.1 diverge holds valuable lessons about AI development philosophies.
In this blog post we’ll cover:
- Background on GPT-4 and Claude 2.1
- Architectural Differences
- Training Methodology Contrasts
- Development Philosophy Divergence
- Use Case Specializations
- Implications for the Future of AI
By examining these key differences we can better comprehend the current AI landscape and where it may progress in years to come.
Background on GPT-4 and Claude 2.1
GPT-4 is the latest installment in OpenAI’s “Generative Pretrained Transformer” series. Originally launched in 2018, GPT models pioneered the pre-training of deep neural networks on vast text corpora to acquire broad linguistic and textual understanding.
GPT-4 specifically is the successor to 2020’s GPT-3, which exploded onto the scene demonstrating an unprecedented depth of language comprehension and generative text capabilities. GPT-4 looks to extend these traits even further, guided by OpenAI’s mission of developing advanced AI systems with increasing safeguards.
In contrast, Claude AI 2.1 comes from startup Anthropic, founded in 2021 to pursue a different approach to language AI development focused on Constitutional AI principles like transparency and controllability. Building off their initial Claude model, Claude 2.1 adds abilities like listening comprehension and summarization while adhering to rigorous self-supervision protocols.
Architectural Differences
While GPT-4 and Claude 2.1 both employ transformer-based neural networks, their architectures have key variations.
GPT-4 inherits GPT-3’s core transformer decoder architecture trained on textual context to predict subsequent text sequences and relationships. GPT-4 expands on this with additional “pathway” modules and trillions more parameters to enhance its knowledge representation abilities.
Claude 2.1 on the other hand utilizes what’s known as an encoder-decoder architecture. The encoder module reads and comprehends textual input, while its paired decoder module learns to generate relevant output text or responses. This explicit input-to-output structure lends more direct control over text generation.
These architectural differences speak to more underlying philosophical divergences in how each system “thinks” about language modeling and processing. GPT-4’s architecture is more implicitly associative, while Claude 2.1 directly maps text inputs to outputs.
Training Methodology Contrasts
GPT-4 and Claude 2.1 likewise exhibit significantly different training methodologies, particularly around human involvement.
OpenAI used a technique called reinforcement learning from human feedback (RLHF) to train GPT-3 on appropriate text generation. GPT-4 expands this exponentially with a wider human feedback program and reinforcement learning models containing over 1 billion parameters. This intensive human involvement helps guide GPT models towards better reasoning and communication skills.
In contrast, Claude 2.1 employs what’s known as “constitutional AI” self-supervision techniques. Rather than relying on human feedback, the Claude model autonomously curates its own training datasets and applies algorithmic self-supervision protocols checking for harmful, unsafe, or inconsistent output. This rigorous self-directed approach aims to produce AI systems with transparent, controllable behavior.
Development Philosophy Divergence
Behind these architectural and methodological differences lies an underlying divergence in AI development philosophies between OpenAI and Anthropic.
OpenAI clearly believes advanced AI can be achieved through sheer scale and heavy human involvement. By expanding model size, datasets, and human feedback mechanisms they aim to incrementally improve systems like GPT-4 towards human levels of reasoning and discourse. The tradeoff is these systems require intensive computational resources and lack self-supervisory safeguards at present.
Anthropic on the other hand thinks advanced AI requires fundamentally different techniques focused on constitutional principles like safety, controllability, and transparency. While leveraging impressive scale as well, Claude adheres to rigorous self-supervision regimes intended to produce safer outcomes with less reliance on human oversight over time.
In effect these firms represent different hypotheses on charting a path to advanced artificial general intelligence. Their innovations display how varied techniques around neural architectures, learning paradigms, and underlying philosophies impact development directions.
Use Case Specializations
Relatedly, GPT-4 and Claude models currently exhibit differences in intended use cases and specializations.
OpenAI bills GPT models as general-purpose text and dialog agents. GPT-4 specifically looks to excel at diverse creative and positional writing while maintaining engaging open-domain conversations. Its foundation as an autoregressive language model lends itself to such broad utility.
The Claude series instead focuses narrowly on technical assistant abilities across areas like information retrieval, classification, semantic parsing, summarization and more. While conversant as well, Claude specializes in bringing structured accuracy to tasks like parsing questions, pulling relevant data, and generating summarized responses.
These use case differences reflect current business objectives and commercialization plans. OpenAI increasingly consumerizes its API and products around GPT abilities, while Anthropic sells Claude-as-a-service to enterprises valuing structured assistant capabilities versus mercurial creativity.
Over time though we can expect both platforms to converge towards similar skillsets with equal parts versatility and expertise. Advanced AGIs will by nature exhibit strong general intelligence alongside specialized precision around valuable domains like research, analytics, and decision support.
Conclusions & Implications for AI’s Future
Reviewing these key developmental differences between GPT-4 and Claude 2.1 carries compelling implications about AI going forward in several regards:
First, it highlights how varied techniques – architectural, methodological and philosophical – can drive progress within the shared goal of human-like intelligence. Testing these divergent approaches expands our toolkit.
Second, it suggests current systems still face distinct capability tradeoffs whether more creative versus structured or generalist versus specialist. Truly balanced, multifunctional AGIs remain on the horizon.
Finally, and perhaps most critically, it proves how ambitions around advanced intelligences must always incorporate constitutional principles and safeguards by design to reach their fullest potential, on both social and technical measures. Integrating safety, controllability and oversight throughout the development process represents a key lesson as artificial general intelligence progresses.
By responsibly leveraging the full stack of human virtues – creativity, empathy, ethics, rationality – perhaps future systems like GPT-5 and Claude 3 can deliver on that greatest promise of AI – augmenting every aspect of human potential for the betterment of all.
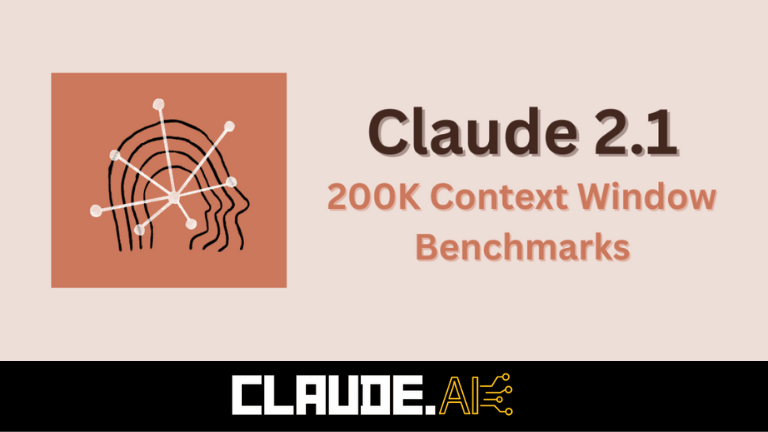