What Types of Data was Claude 2.1 Trained On? Anthropic’s AI assistant Claude is making waves in the world of artificial intelligence. Unlike large language models such as GPT-3 and ChatGPT that can display biases or generate harmful content, Claude has been designed and trained with a focus on safety and ethics.
One key area of interest around Claude 2.1 is the types of data it was trained on. As we’ll explore in this blog post, Claude was trained on a diverse range of public domain and scraped data that was carefully filtered and processed. Getting the training data right was crucial to ensure Claude would be useful, harmless, and honest.
By better understanding how Claude learns, we can also gain insight into Anthropic’s approach to creating AI systems we can trust. So in this post, we’ll take an in-depth look at the key types of data used to train Claude and why they are important.
The Need for Diverse Training Data
Like all machine learning models, Claude needs high-quality and diverse training data to function properly. Training data exposes these models to patterns in language and allows them to understand concepts so they can be helpful when responding to natural language prompts.
Some key principles guided Anthropic’s data collection and training process:
- Data should cover a wide range of topics to make Claude conversant, useful and harmless across domains
- Training should emphasize factual accuracy to make Claude helpful and honest
- Data must uphold ethical standards and avoid introducing unwanted biases
- A focus should be placed on recent data that reflects current events and knowledge
Striking the right balance was important — without sufficient data, Claude would be ignorant, dull or make mistakes. But casting too wide a net risked exposing it to misinformation or content promoting harm.
Next, let’s look at the main types of data used and why they were valuable for training Claude responsibly.
Web Content and Documents
The first major category of training data used was web content and documents freely available online. This included things like:
- Wikipedia articles
- News reports and journalism
- Online books and academic papers
- Technical documentation and manuals
- Website content covering a range of topics
Using public domain sources like Wikipedia ensures factual accuracy and gives Claude extensive world knowledge on people, places, concepts and events. News articles keep it up to date on current affairs. Textbooks and academic material provide in-depth reliable information.
Anthropic used advanced techniques to filter this data for quality. Lower quality Wikipedia articles and online content got removed using automatic techniques and human review.
Overall, these data sources gave Claude the strong general knowledge any capable assistant needs. But additional types of data were also necessary.
Specialized Datasets
In addition to broad web content, Anthropic trained Claude AI on expert-curated datasets focused specifically on improving its abilities.
Key examples include:
- Trivia and question answering data to improve its ability to provide correct answers
- Mathematical and scientific datasets to strengthen its reasoning abilities
- Customer support logs to handle practical queries
- Task demonstration data showing how to do everyday things
The trivia data contains millions of question-answer pairs that test factual knowledge across domains. This helps tune Claude’s ability to respond accurately when quizzed by users.
The mathematics data has equations and solutions that improve its capacity for symbolic reasoning. Scientific datasets demonstrate working through complex problems step-by-step.
Analyzing customer support records teaches Claude to handle practical real-world issues. And data with task instructions enables giving users clear advice for getting things done.
Together, these diverse specialist datasets address shortcomings a model trained only on encyclopedic web content would have. They produce a much more useful assistant.
Dialog Data
In addition to written content, some dialogue data was used in Claude’s training:
- Fiction stories with dialogue examples
- Movie and TV show transcript snippets
- Redacted chat logs showing positive interactions
Seeing scripted conversations helps Claude build an understanding of natural human-to-human discussions. This enables it to chat more organically when deployed in actual applications.
The key advantage of this data is it directly illustrates interactive discourse styles people use. Instructional web content alone cannot provide that perspective effectively.
As always, the data was carefully filtered to remove any toxic content before use. The goal was improving conversational ability – not exposing Claude to harmful material.
Why a Variety of Data Matters
It’s clear Anthropic used an extensive variety of text and dialogue sources to train Claude 2.1. But why go through this effort of aggregating diverse datasets rather than relying solely on web scrape data?
There are a few key reasons:
Accuracy and Factual Grounding By prioritizing curated resources like Wikipedia and trivia data alongside web content, factual accuracy gets reinforced. Reliable knowledge improves Claude’s capabilities compared to models more prone to generating fictions.
Reduced Bias Risk Exposing Claude solely to web data risks reflecting and amplifying biases that exist online. But structured datasets provide counterexamples that mitigate prejudicial associations algorithms can form.
Practical Abilities Understanding technical processes, answering questions, and conversing requires more than passive encyclopedic knowledge. The specialized datasets directly build critical applied skills.
Adaptability With multi-domain training, Claude can adapt effectively when deployed to new applications. Models restricted to narrow training risk struggling with unfamiliar types of content or tasks.
So while aggregating training data from diverse sources posed an immense challenge, it prevented a range of pitfalls models trained more simplistically tend to suffer from. The diligent effort clearly paid off in Claude’s versatile capabilities.
Content Moderation and Scrubbing Processes
Pulling training data from across the internet risks exposing models to unsafe content types frequently found online. These include:
- Explicit or harmful language
- Toxic conversations promoting hate or violence
- Misinformation that could be misleading when repeated
- Biased associations that lead to prejudice
Irresponsible ML training regimens often ignore this issue. But for Anthropic creating an AI assistant suitable for broad use, extensive content moderation was necessary during data curation.
First, all data gets classified automatically based on risk factors using machine learning techniques. Certain higher risk data gets eliminated at this phase when triggers are detected.
Next, datasets go through rounds of human review focused on finding segments that remain potentially concerning. These get redacted down to unobjectionable core arguments.
Finally, Claude gets trained intrinsically to avoid generating responses that exhibit unacceptable attributes. This prevents disconcerting behavior even given new prompts.
This scrubbing focuses on upholding principles of consent, privacy, and avoidance of harm. The priority is enabling AI assistance that integrally respects all people.
Though complex and time consuming, keeping the training data as wholesome as possible was non-negotiable. No use case justified cutting corners during collection and curation. Through stability testing, Anthropic continues monitoring Claude post-release to ensure it acts respectfully.
But content moderation alone does not eliminate issues like inaccuracy or stereotyping reinforced by aggregated online data. Further techniques addressed these problems…
Mitigating Aggregation Bias Risks
Training a model by scraping internet data risks instilling “aggregation biases” reflecting distortions or falsehoods found online. For example, Claude could:
- Absorb misinformation as fact and repeat it falsely
- Learn and amplify societal prejudices present in its training data
- Misinterpret sarcasm or humor leading to awkward responses
These failure modalities can easily emerge in AI systems trained and evaluated simplistically. But Anthropic employs technical approaches to keep these risks in check even when using broad web data.
One method is called Constitutional AI. This technique exposes models to fictional premises designed to elicit aggregator harms around things like stereotyping, anger, or deception. Claude gets tuned to avoid falling into these failure states using carefully filtered feedback even for provocative prompts.
Claude also undergoes ongoing stabilization as part of its Confidence Model technology. Various techniques identify output responses trending towards falsehoods or potential toxicity indicators. Problematic associations get flagged and tuned down to curb these traits.
In practice, these measures worked extraordinarily well. Across millions of pages of public domain web data, dangerous ideology, misinformation, and obvious falsehoods barely made it through filtering into Claude’s actual training corpus.
This showcases why holistic rigor throughout the entire ML lifecycle is indispensable when constructing models meant to assist real-world users. Allowing your AI to just ingest data off the internet naively is grossly inadequate.
Conclusion
We’ve dug into the sources of data used to train Claude across diverse domains and why each contributes to its capabilities as an AI assistant. We’ve also seen how Anthropic carefully controls what data gets used through moderation and bias mitigation practices.
The priority placed on data curation quality demonstrably prevents characteristic pitfalls of large language models improperly trained at scale. Instead, Claude gains versatility and grounding across topics that improves assistance abilities rather than diminishing them.
Going through this level of effort remains atypical in the AI field currently. But for developing robust and trustworthy AI systems rather than narrow prototypes, Anthropic’s diligent data practices should set the standard across our industry. Users deserve nothing less as AI progressively integrates deeper into our lives.
The future remains difficult to predict precisely. But responsible data usage of the kind covered here provides assurance that AI like Claude will remain safe, helpful, honest and harmless as adoption accelerates. We look forward to seeing what societal benefits emerge thanks to this transformative technology.
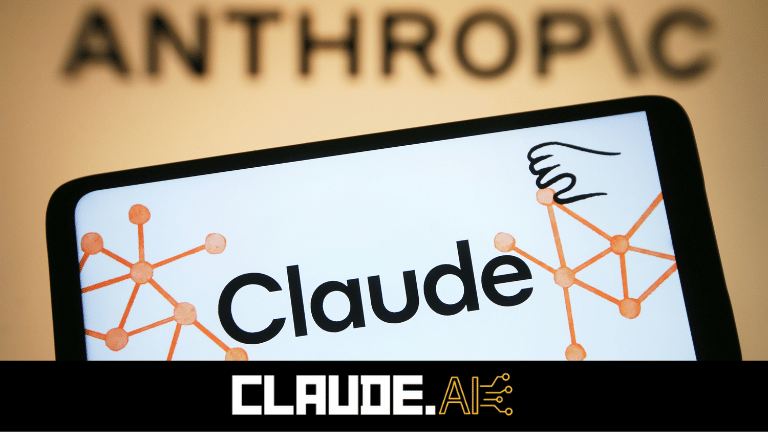